“Data is the new oil,” they said…in like 2015. But what about 2023? According to an analysis by the European Commission, the value of the data economy is predicted to exceed €1 trillion by 2025 for Europe alone. The demand for data, especially good data, is poised to grow: the number of companies using generative AI products has increased by 1310% from November 2022 to May 2023, but these models often require high-quality data in order to perform effectively. And good data is difficult to source, clean, and structure.
Synthetic data can play a significant role in AI model training and development, but also in many other data science use cases, while addressing security requirements along the way.
This article will dive into the following topics:
- What synthetic data is and where it fits into the AI and data landscape
- The key drivers of synthetic data adoption
- The current market landscape for synthetic data
- Opportunities and takeaways for investors and entrepreneurs
What is Synthetic Data?
Synthetic data refers to data that is artificially generated from computer simulations or algorithms, which reflects the characteristics of real-world data. Synthetic data can be created with or without a prior reference dataset. When using a real-world dataset as its reference, the synthetic data preserves the original statistical relationships between items, but generates new observations that also remove sensitive information.
For example, if a financial services company has databases of customer transaction data, it could create synthetic versions that contain the same patterns as the original dataset, but protects sensitive information by modifying personal identifiers such as names, birthdates, etc.
To create synthetic data without any reference data, users can specify certain features or parameters, and then use a simulation to generate data points. This method has been used to create images of roads in different weather and lighting conditions, for instance, in order to train autonomous vehicles in a variety of situations.
What kinds of data can be synthesised?
- Structured data is essentially tabular data, with rows and columns, and a predetermined format. This can include databases with text, numerical data, time series, event data, etc.
- Unstructured data includes images, audio, videos, 3D assets, text that are stored in their original format, without any predefined order or schema.
Why do we need synthetic data?
While there are many uses of synthetic data, there are four main drivers of enterprise adoption:
- Cost: Collecting and processing relevant, real-world data into usable formats is extremely costly. It is estimated that 80% of data scientists’ time is spent cleaning, collecting, and preparing data. Enterprises spend significant amounts of time and money on gaining access or collecting the right data, labeling/annotating it, and validating it for their use cases. This often involves fragmented tools that do not fully address potential problems such as dataset bias. In some cases, such as sensor-based data collection (eg. for computer vision), the data collection process has to be repeated from scratch if the sensor configurations change. Synthetic data, on the other hand, is cost-effective for generating useful, labeled datasets, especially for unstructured data collection.
- Volume: Even if enterprises have existing data, they may not have enough of it for their use case. Synthetic data is extremely effective at providing accurate datasets at scale, by generating endless variations of labeled data. For example, in the case of medical imaging data, datasets are often small or difficult to access due to regulations. However, even relying on a small sample of original data can allow synthetic data methods to generate large volumes of usable imaging data.
- Privacy: Enterprises in highly-regulated industries, such as government, healthcare, and financial services have strict laws regarding data privacy, usage, and access. So even organizations that have all the data they need may be unable to truly get value from it. Synthetic data can play a role, by preserving the structure of the original data, but changing the sensitive information, allowing organizations to use it for software testing, vendor collaborations, business analytics, and more.
- Bias: Companies are also turning to synthetic data to fix imbalance and biased datasets. For example, to create its AR face filters, Snap estimated that it would need data on 70,000 different identities to ensure the filters could adapt to a diverse range of faces, body features, and characteristics. Manually collecting, labeling, and processing this data would be impossible. But by generating its own synthetic data pipeline to train the ML models, the company was able to create filters to be used at scale.
The Synthetic data landscape
Synthetic data is still an emerging space, with the majority of existing companies founded in 2021 or 2022, and a few in 2023. According to PitchBook, there are an estimated 131 synthetic data companies (with synthetic data as their primary offering) currently operating the market, but that figure continues to grow.
Of the pure synthetic data players, most of these companies (68) operate in structured synthetic data, and key industries include healthcare, financial services, automotive and robotics. This does not include companies that are in stealth mode or have been acquired.
Note: The data mentioned and depicted in the charts is based on data sourced from Pitchbook, Crunchbase, and LinkedIn, and may have errors due to incorrect labeling or missing data points.
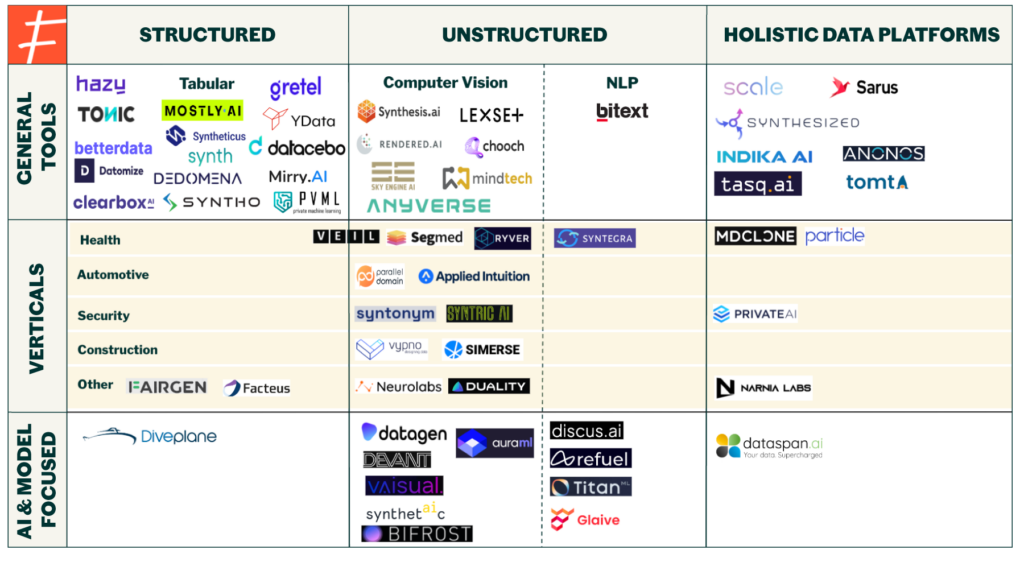
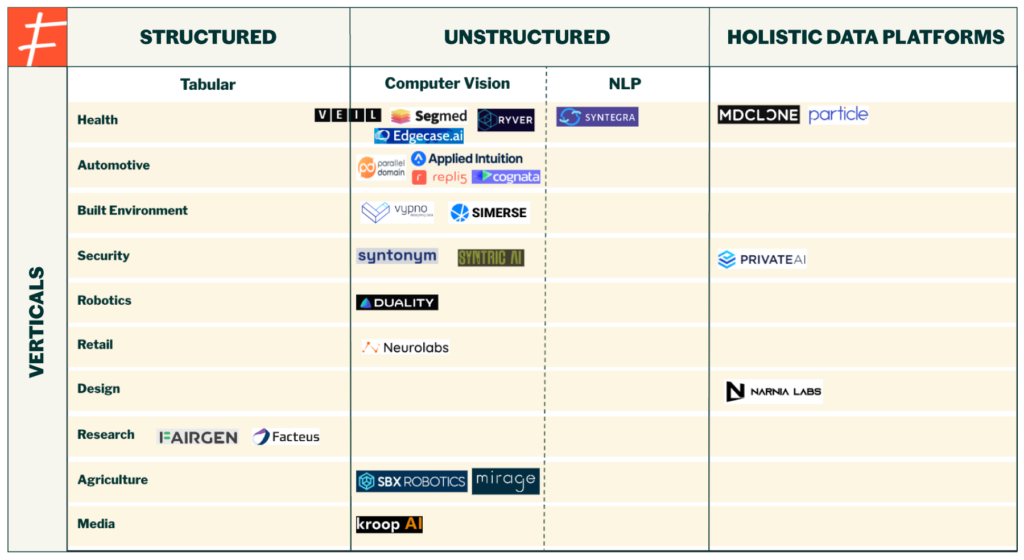
Part I: Structured Data
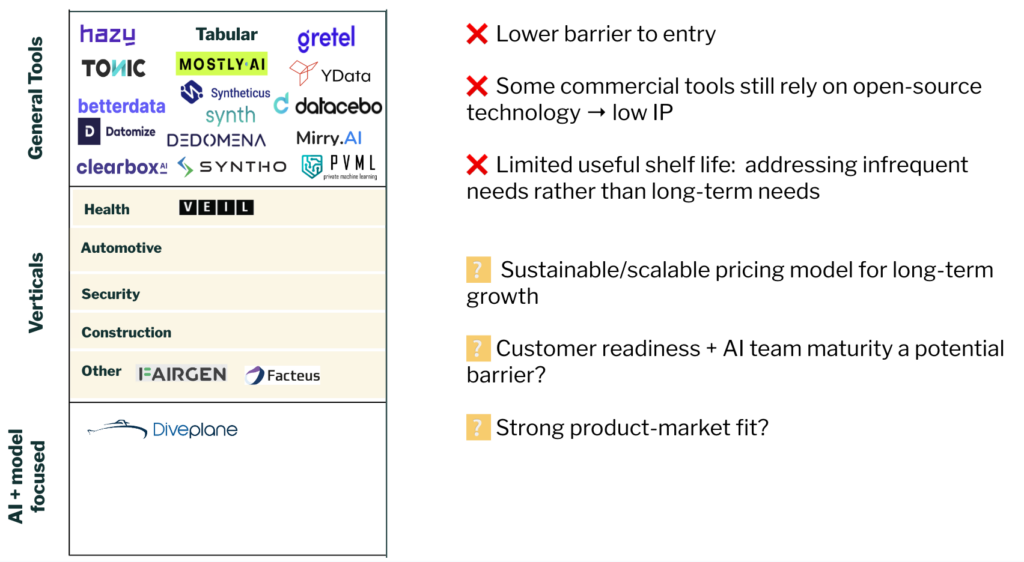
The structured data segment is perhaps the most crowded area of the synthetic data market — the companies depicted in the general tools section only represent a fraction of the companies providing tabular data. These vendors predominantly target highly-regulated industries such as healthcare, financial services, telecomms, and government, where customers have significant amounts of data, but face difficulties with data privacy and compliance requirements. Synthetic versions of existing data therefore allows these companies to get use of their datasets while avoiding severe fines and consequences tied to breaching strict regulations.
For example, personally identifiable information can be replaced and then shared throughout the organization for analytics purposes, software testing, marketing, AI development, and more. These platforms are geared towards developers, but some are also meant to be used by technical and non-technical users alike.
However, even though privacy is the main value proposition advertised by these companies, it is unlikely that privacy will ultimately be a primary driver of adoption for enterprises. This is because privacy-preserving synthetic data tends to be a business need, while most platforms are focused on developers. In addition, these platforms address one-time or infrequent needs, which would not justify the use of a standalone synthetic data platform for tabular data.
Cases such as software testing, meanwhile, require continuously changing datasets rather than a one-time snapshot. And whilst they would greatly benefit from synthesized copies, synthetic data has not gained as much traction here as expected, likely due to the amount that enterprises spend on this area in the first place. Another possible explanation is that enterprises in this area, such as J.P Morgan, are developing those capabilities internally, which poses a question around market size: will these verticals be big enough for tabular companies to supply synthetic data to, given that some enterprises are building in-house?
Other enterprises are still relying on legacy masking and anonymization techniques to address data privacy concerns, which are not fit for purpose as they can be reverse-engineered. They seem slower to adopt synthetic data solutions, even though they would be a better alternative, which prompts questions around customer readiness and AI team maturity, and whether it is a barrier to synthetic data adoption in the short term.
Other challenges for structured synthetic data companies lie in developing a sustainable and scalable business model, given that many are currently creating on-demand datasets for non-recurring usage. One interesting trend is that platforms in this area are becoming more horizontal and are eventually seeking to target multiple users, from developers to business analysts. This, along with competition from broader data management platforms that offer structured synthetic data as a feature, means there is likely to be consolidation in this area.
Part II: Unstructured Data
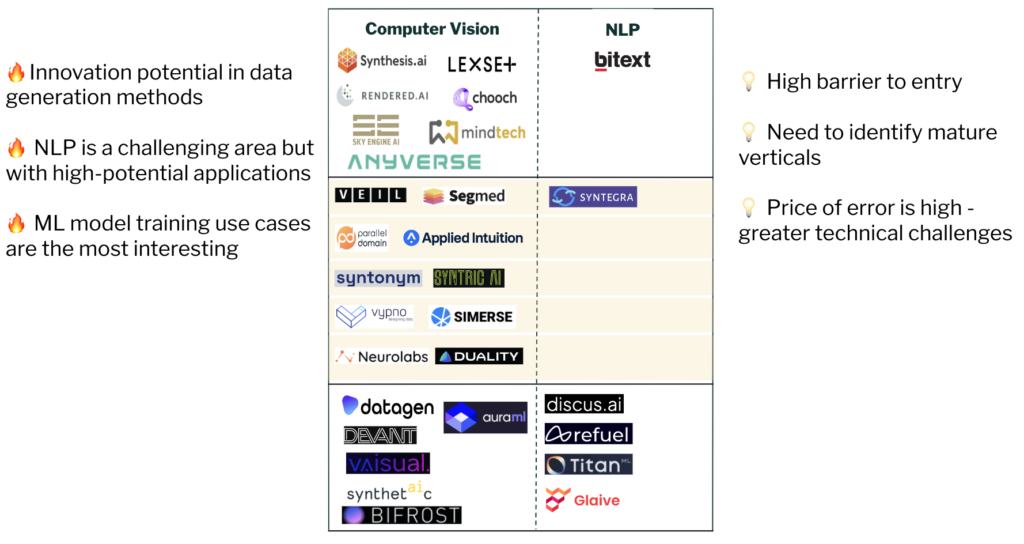
Unstructured synthetic data is one of the most fascinating segments of the landscape. Currently, many companies are working with images in computer vision applications, such as edge cases in autonomous vehicles (driver monitoring, pedestrian detection, on and off-road autonomy), geospatial-related use cases in security and defense, aerial vehicles, search and rescue, and VR use cases such as virtual try-on applications in retail.
Synthetic data technologies are also being used at the application layer, by companies such as Respeecher for voice cloning and Synthesia for video. Use cases include creating deepfakes for the film industry, and advanced biometrics for contactless payments systems.
Synthetic data for natural language processing (NLP) is another high potential area, especially as high-quality training data can be limited for many use cases and languages. Systems like Amazon’s Alexa were trained on synthetic data for natural language understanding tasks, where researchers relied upon a few rich speech samples that they then used to train synthetic data generation models, in order to produce similar content based on the grammatical rules learned from the existing data. While more recent advances show how natural language understanding (NLU) systems can “learn” new accents with very little training data, these cases still rely on training existing foundation models in English. Synthetic data can, therefore, play a significant role in building foundation models even for low resource languages with limited corpora, and provide smaller, high-quality datasets for fine-tuning . Companies such as Refuel, Titan, and Glaive are just now starting to explore this, with more ventures likely to follow.
Unstructured synthetic data can also provide training data for ML models where traditional data collection is insufficient, impossible, and/or difficult to carry out at scale. But, there is a higher barrier to entry for this segment since vendors often deal with the technical challenges of specific verticals, and complex data generation methods.
Vendors in this area need to identify use cases with an immediate and continuous need for data, as there are a wealth of opportunities not only in the synthetic data infrastructure layer, but also in synthetic media applications.
Part III: Holistic Data Platforms
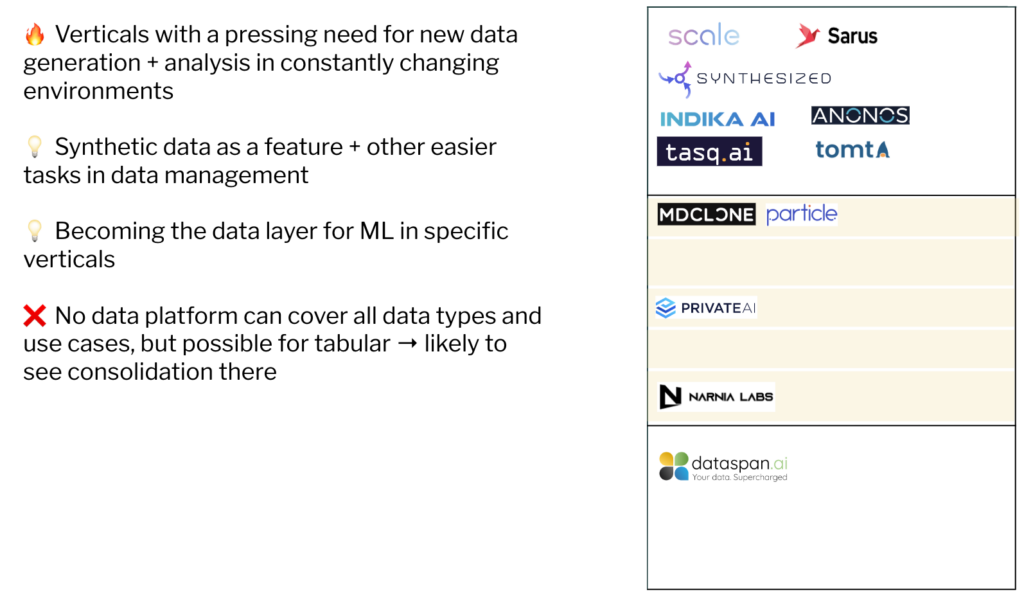
Current Market Dynamics
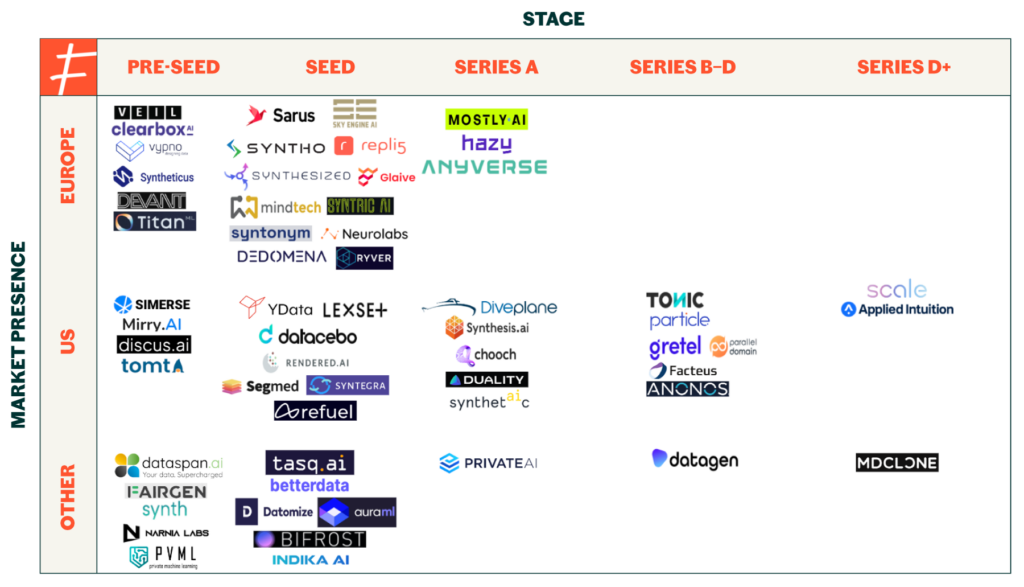
Even though synthetic data has only gained popularity in recent years, there have already been a few exits, such as that of Replica Analytics (acquired by Aetion), and AI.Reverie (acquired by Meta).
As AI gains more traction and enterprises make serious efforts to deploy their own ML models, the quiet industry of synthetic data is becoming ever louder. Interesting early-stage include: Hazy ($6.4m) in 2020, Datagen’s Series A ($15m), Parallel Domain ($11m) in 2021, Bifrost ($5.1m) in 2022 and Betterdata ($1.65m) in 2023. Interesting growth deals included Scale AI’s Series C ($325m), MDClone’s Series C ($66.5m), and Mostly AI’s Series B ($25m) in 2021 and 2022, and Hazy ($9.38m) and Cognata ($4.3m) in 2023.
Synthetic data and the rise of AI
So far we’ve seen why enterprises are starting to turn to synthetic data solutions, but as technology advances there will be many other long-term, enduring problems that synthetic data can solve.
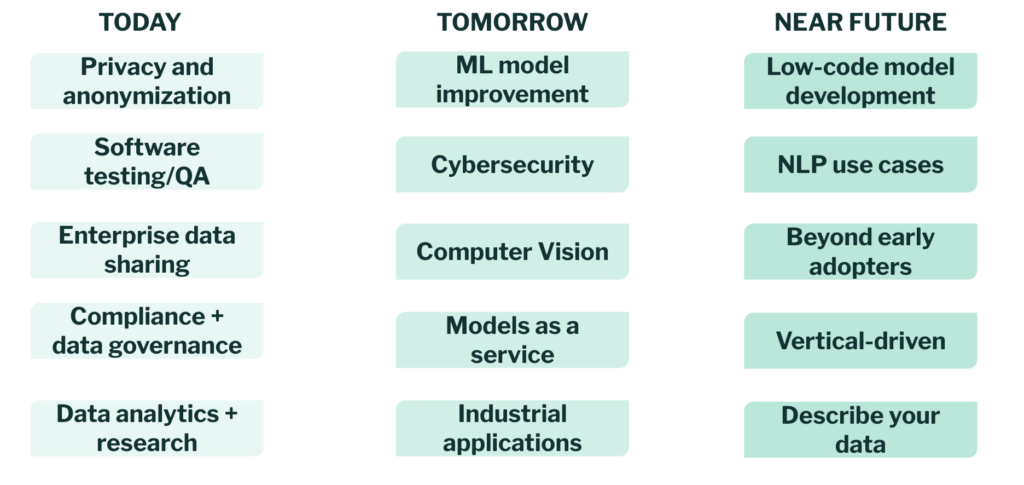
Despite a wave of synthetic data companies appearing in the past few years, the market is still in its early stages. There is a growing proposition for data-centric AI, and synthetic data can power this new data engine for AI.
Synthetic data has significant potential to accelerate, scale, and enable ML production and deployment, but this depends on a few factors such as identifying immediately valuable use cases, a sustainable business model, and integrating synthetic data with the rest of data operations for ML model development.
Additionally, there are areas outside of AI development where synthetic data can power transformative applications, such as security (including deepfake detection, penetration testing), applications for environments such as industrial settings, as well as low-code applications.
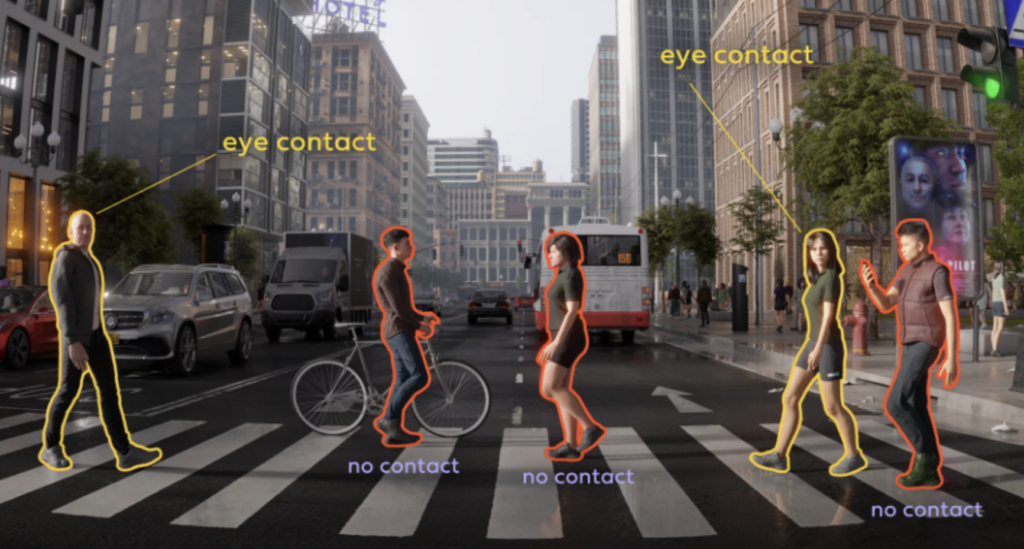
Key opportunities and takeaways
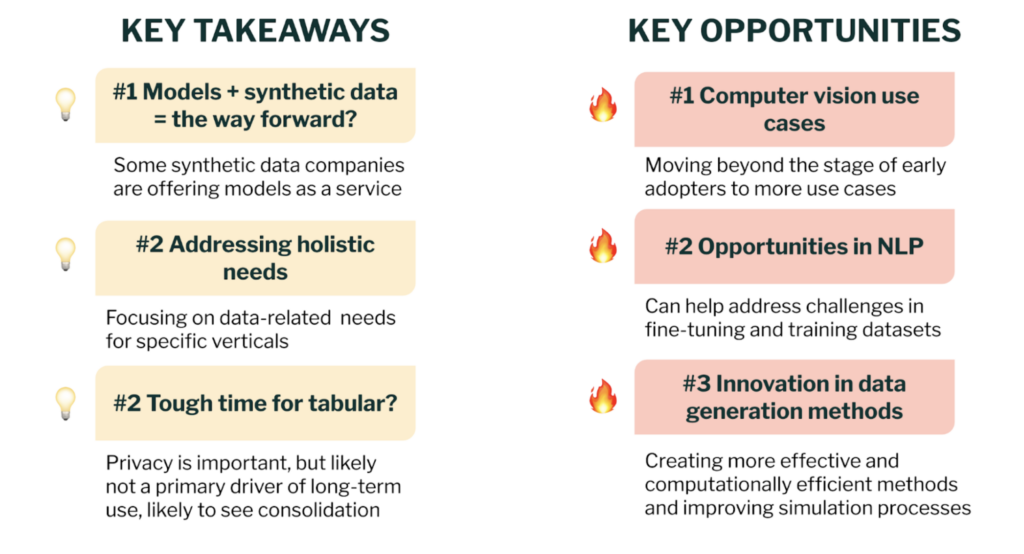
Key Takeaways
#1 Synthetic data vendors can train their own models, too
Providing synthetic data alone might not always cut it. Companies that also provide their own foundation models trained on synthetic data, for the customer to potentially fine-tune on real-world data, will likely capture more value as enterprises seek to implement AI as a core capability, rather than an experimental initiative.
Companies providing the right data and base models that enterprises can adapt can allow enterprises to productionize ML models faster than before.
#2 Addressing vertical-specific data management lifecycles
Specialized synthetic data companies owning the end-to-end data pipeline will likely gain more traction in specific verticals. Rather than meeting infrequent requests for datasets, and leaving customers to figure out the rest on their own, companies can address the entire data-for-AI process which not only includes dataset creation, but also annotation, post-processing, and dataset curation for post-model deployment.
This can also include building out the ecosystem of relevant tools, such as sensors and digital twins, in the case of Duality, or integration with hardware for real-time data ingestion and processing. It is unlikely that one vendor will ultimately cover all verticals, data types, and use cases, simply because industry-specific requirements and challenges with different data types are too vast.
#3 Tabular data space will likely see consolidation
While tabular synthetic data companies emerged from 2018, most of them have still faced challenges with product-market fit. The main value proposition they offer is around privacy-preserving data, however, there are some challenges around business model, budget, spend, and infrequent use in enterprises, suggesting that there will be limited long-term growth for standalone structured data providers (Anonos’ acquisition of Statice also highlights this, as well as Apica’s acquisition of Logiq for data observability).
While regulations such as GDPR underline the importance of data privacy, it might not be a primary driver of adoption, as synthetic data is mainly used by developers. Also, many of the large enterprises that these vendors target tend to have internal resources to build in-house. Even if they do not, structured data vendors tend to secure one-off contracts or end up in a consulting-style arrangement, rather than seeing recurring usage.
Key Opportunities
#1 Computer vision use cases
Computer vision use cases are fascinating but also present a variety of challenges due to the simulation-to-reality gap (but recent advances show progress).
However, as data generation methods continue to evolve, computer vision data can significantly fill the need for data in areas such as robotic control and perception, industrial automation, and contexts where input data is constantly changing yet needs to be analyzed and dealt with in real-time. Vendors in this area are still working with early adopters in healthcare, robotics, construction, defense and security, manufacturing, industrial settings, and agriculture and forestry. Such use cases would require deep technical knowledge, and presents an opportunity for specialized technical players to come out on top by capturing a large swath of the market.
#2 NLP
A few companies have recently emerged to provide LLM-generated synthetic data, such as Discus AI and Refuel, but this area is still a nascent one. Language models face well-documented problems including bias, hallucinations, and output reliability, this is an area where synthetic data can potentially play a role. For example, while language models are still good for generic themes, they do not capture nuances in semantics that are important in domains such as legal. Synthetic data usage in this area can be complemented by external knowledge bases to ensure thorough evaluation of the data, presenting a ripe area of opportunity to generate high-quality input for language models.
There are also few non-English models being developed. A few initiatives are underway to build LLMs for Arabic, Chinese, and a few other non-English (mostly European) languages, but synthetic data can contribute to foundation models in low-resource languages. While different methods have been proposed, such as unsupervised prompt engineering for low-resource languages, synthetic data for NLP can potentially address these problems at scale.
#3 Innovation in data generation methods
There are currently trade-offs between different synthetic data generation methods, especially for metrics measuring quality, fidelity, and scalability. Innovation in data generation methods, however, can address these problems by improving the simulation to reality gap, sample diversity and data balancing, data quality, model collapse, and computational processing power required for each approach.
Advances in multimodal data generation and integrating human expertise, photorealistic game engines and diffusion models (especially for image generation and LLM-enhanced diffusion models) show that there are still opportunities for innovation in methods to generate synthetic data. Additionally, automating the rendering and simulation process through programmable AI tools can improve the simulation approach for synthesizing data, as vendors currently rely on game engines.
Synthetic data ultimately fills big gaps that current methods cannot address, especially high-quality and novel data generation, which are still pressing problems, especially for engineers in need of large unstructured datasets. Therefore, new data generation methods can completely change the game.
CONCLUSION: BECOMING AN INDISPENSABLE PART OF THE AI STACK
There’s no question about it — data is essential to power AI models, and synthetic data will be tied to the rise of AI. But other questions are also emerging around data pipelines, such observability and monitoring, dataset evaluations, etc. Expanding into other areas, whether it is data curation, testing and evaluation, model management and validation, can allow more value to be obtained from synthetic data, especially if the target domains are easier to enter.
Evaluating datasets is becoming extremely important in ML observability, and integrating synthetic data offerings into this broader space can also helps address issues such as bias, explainability, and accuracy over the course of ML model development, as well as data monitoring and anomaly detection, such as alerting developers for hallucinations, etc.
Even in the case of synthetic media applications, compliance and safeguards still remain an issue. Some major data management companies are not compliant with major industry regulations (such as in healthcare) and other synthetic media companies have faced serious security breaches, allowing bad actors to gain access to their sensitive technologies. But, synthetic data companies must integrate their offerings into the right ecosystem of tools and methods for their use case/industry, and link outcomes to specific ROI and goals within the enterprise, such as putting X number of models into production, and generating clear cost and time savings for ML engineers.
The potential of synthetic data in AI tooling and applications also calls into question the defensibility of data moats. While companies will still have proprietary customer data that their models can ingest and learn from in real-time, the accessibility of synthetic data suggests that data might not be a strong differentiator in the early-stages anymore. Data used to be considered the “new oil”, but synthetic data allows everyone to create their own wells of oil. Now, when all engineers have the ability to create unique, precise, and highly customizable datasets for particular use cases, companies will have to devote more attention to outperforming on metrics for model performance, rather than relying on the fact that their unique data gives them an “edge”.
While data moats can become deeper (and more difficult to overcome) as a company gains more customers — and thus access to exclusive data — synthetic data will make differentiation harder in the beginning, forcing companies to build more robust and innovative technologies.
When it comes to AI, even building a technological moat may not be that easy, given that designing an algorithm that outperforms even open-source ones using the same data is difficult. Companies will need to pay more attention to how they can increase their defensibility, as synthetic data will democratize access to high-quality and diverse data. It will likely be more important for companies to deeply understand customer needs and how their AI application can become a key part of that workflow, focus on speed, or differentiation in sales or strategy, in order to get ahead of the competition. Or, it might be that stellar technical teams will matter most, and will be the foundation of an AI moat in the future.
Acknowledgements
Thank you to Sergey Toporov, Akash Bajwa, Danel Dayan, Sebastian Dziadzio, Emanuele Haerens, Niccolò Comparini, Kathrin Khadra, Miguel Martinez, Ofer Lavi, Viktar Atliha, Anna Kovalenko, Alex Liu, Brian Donovan, Khush Gupta, Maxime Agostini, Nicolai Baldin, Olivia Tomic, Steven Atneosen, Uzair Javaid
and to Ruth Sheridan, William McQuillan, Hannah Skingle, and the rest of the Frontline Ventures team.
Links and further reading
- Synthetic Data Could be Better than Real Data – Nature
- The importance of synthetic data in data-centric AI – Fabiana Clemente
- Why synthetic data can be useful in machine learning – MIT
- Potential of synthetic data for videos – Kim et. al 2022
More on synthetic data generation methods
- Synthetic tabular data generation – Fabiana Clemente
- Overview of Diffusion models, GAN, VAE – Lilian Weng
- Diffusion models vs GANS – Dhariwal & Nichol 2021
- VAE vs GAN – Xuandong Xu
- More on generative methods – Bond-Taylor et. al 2022
- Tabular data with GANs – Casper Hogenboom
- Generating images with GANs – NVIDIA Research
Special shout-out to the data-centric AI community!