As AI startups gain traction at an unprecedented pace, investors face a new set of challenges in distinguishing what characteristics are indicative of future category leaders, versus mere hype.
Unlike traditional SaaS, we expect AI-first applications to thrive with different business models, growth levers, and defensibility factors.
In this second part of our series on application-layer AI, I lay out five essential questions that investors must ask to successfully navigate this evolving landscape. See part one for our five essential questions for application layer founders.
1. Is traction becoming a distraction?
AI companies can generate revenue much quicker than traditional software companies. They can essentially bypass the product-market-fit stage (PMF) stage of building a company if they are simply automating existing workflows or services work with immediate value-add. Additionally, as many enterprises are keen to explore the potential of AI, they have dedicated experimental and innovation budgets to try out these solutions. Getting €50k ACV from these customers for experimentation is very different from an at-scale, recurring contract with the product embedded in core operating work-flows. As a result, AI startups are gaining traction faster than ever. For example, Cursor went from $1M ARR in 2023 to $65M ARR by Nov 2024.
Many of these AI companies operate like mini “agencies” rather than software companies. We could end up with hundreds of these agencies at €1-10m ARR, as they each have an ability to win work quickly in local markets and for specific use cases (more on that later). As a result, getting to €500k-1m in ARR at seed stage doesn’t really tell you much as an investor. It provides some early validation of ability to sell, but in the journey of becoming a €1B+ valuation company it is a rounding error. For these “agency”-like agentic companies, the revenue is very undifferentiated and un-sticky, making it quick to acquire and quick to lose.
Implications for Investors:
Investors must see through revenue metrics and assess the business on the other facets discussed in part one e.g. how opinionated is the product, access to differentiated data, re-imagining existing workflows etc. These facets are going to define who becomes the category winner more than early revenue, particularly if that revenue is experimental or proof-of-concept based.
2. What type of AI and what level of automation does the product unlock?
Not all AI is created equal, and it’s important to distinguish the category of AI being developed, as well as the depth of innovation. Broadly, there are three types of AI product:
- Co-pilots
- Agents
- AI embedded in services
However, it’s important to go one step deeper. Even for “agentic” solutions that are autonomously executing on tasks, there are still nuances in the level of automation to which they operate. Langchain provides a useful five level framework for agentic automation, distinguishing between whether code (written by a human) or an LLM are executing on identifying the steps to take, choosing which step to take, and generating the output. Different levels of automation may make sense for different product and differentiation strategies.
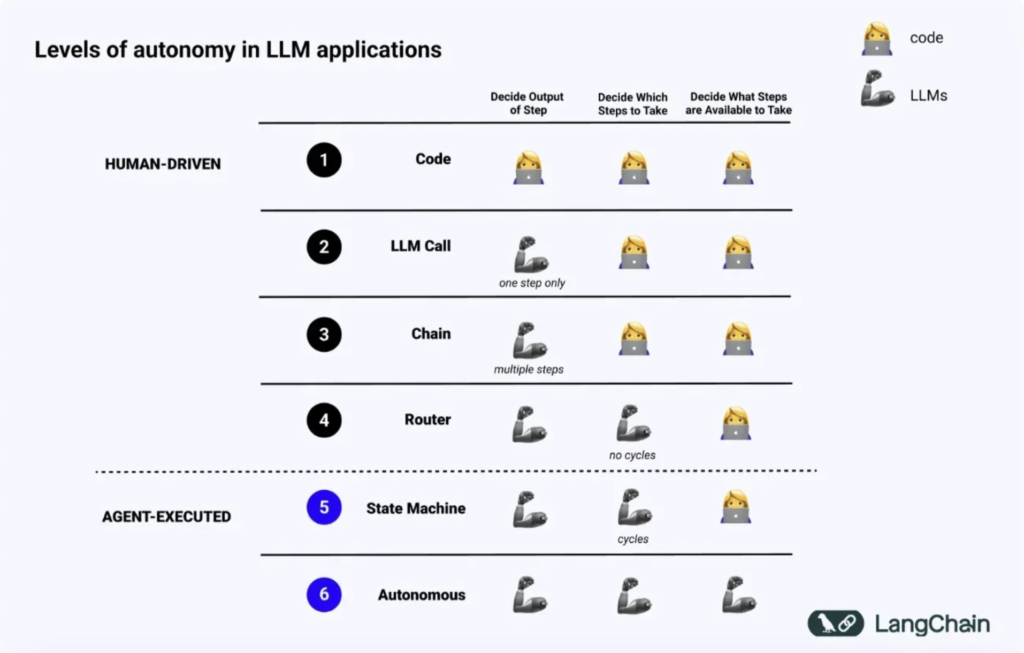
For example, Github copilot is trying to own level 2 automation by suggesting code but giving developers power to make final decisions; UIPath thrives at level 4, with agents handling repetitive tasks but escalating to human in the loop for any issues; Wayve technologies is pushing for full level 6 autonomy through its end-to-end deep learning for AVs.
Implications for Investors:
Take the time to truly understand the type of AI at work, and the level of automation at play for every company assessed. On the surface level they may look the same, but each step towards level 5 automation is a step-change in technical sophistication and product positioning.
3. What role do professional services firms have to play?
As large enterprises work out how to extract value from AI and deploy it in a responsible and secure way, they are increasingly turning to consultants for guidance and implementation support. Professional services firms will therefore play a greater role earlier on in an AI startup’s lifecycle than in a traditional SaaS lifecycle, supporting with custom AI integration, build tailored use cases, and at-scale deployments. Accenture, for example, secured $3B in generative AI bookings in 2024, illustrating the immediate revenue opportunity for consultants.
This may seem counterintuitive as AI has the potential to erode the long-term revenue base of professional services firms. We expect to see a short term boost to services revenue, but over time we expect there to be two types of category winner emerge:
- Tech-enabled services firms, with business models optimized for AI that will disrupt service incumbents through offering outcomes that are better, faster, and cheaper.
- AI software platform companies that fully productise the repeatable use cases that are emerging from bespoke services projects – just as ERPs, CRMs, and HRIMs emerged in the past
Over time, enterprises will shift from bespoke AI solutions and instead outsource to either software platforms or tech-enabled services, as a way to reduce operating complexity and drive competitive advantage.
Implications for Investors:
We expect to see professional services play a large and early role as channel and integration partners for AI startups. Investors need to distinguish between those that may remain as tech-enabled managed service providers, and those that can evolve into standalone platforms that disrupt the services industry.
4. Is this company embracing new business models?
New platform shifts lead to a refresh of optimal business models. For example, legacy on-premise software lent itself to perpetual licences, while the shift to cloud computing drove the adoption of per-seat pricing. Established companies often struggle to adapt their business models to new paradigms, as doing so can erode their profitability structure. This offers new entrants, optimised for new business models, an advantage over incumbents.
For SaaS products, per-seat pricing makes sense because users interact directly with the software. In this model, revenue scales with the number of users—an early form of usage-based pricing. AI-driven applications, however, can deliver value that is completely decoupled from the number of seats (people) interacting with it. Agentic AI systems perform tasks autonomously, often without a human in the loop, and their costs scale with usage—every time they need to ping an LLM API for the customer request. If a company continues charging per seat while its costs are driven by usage, it risks a margin squeeze.
At the same time, customers increasingly expect to pay based on actual value received rather than flat fees. Together, these trends point to a shift towards outcome- or value-based pricing models. We also anticipate a growing ecosystem of tech companies that enable this shift by powering dynamic, usage-based pricing (e.g. Metronome). Here is a list of some of these business models we are seeing in market:
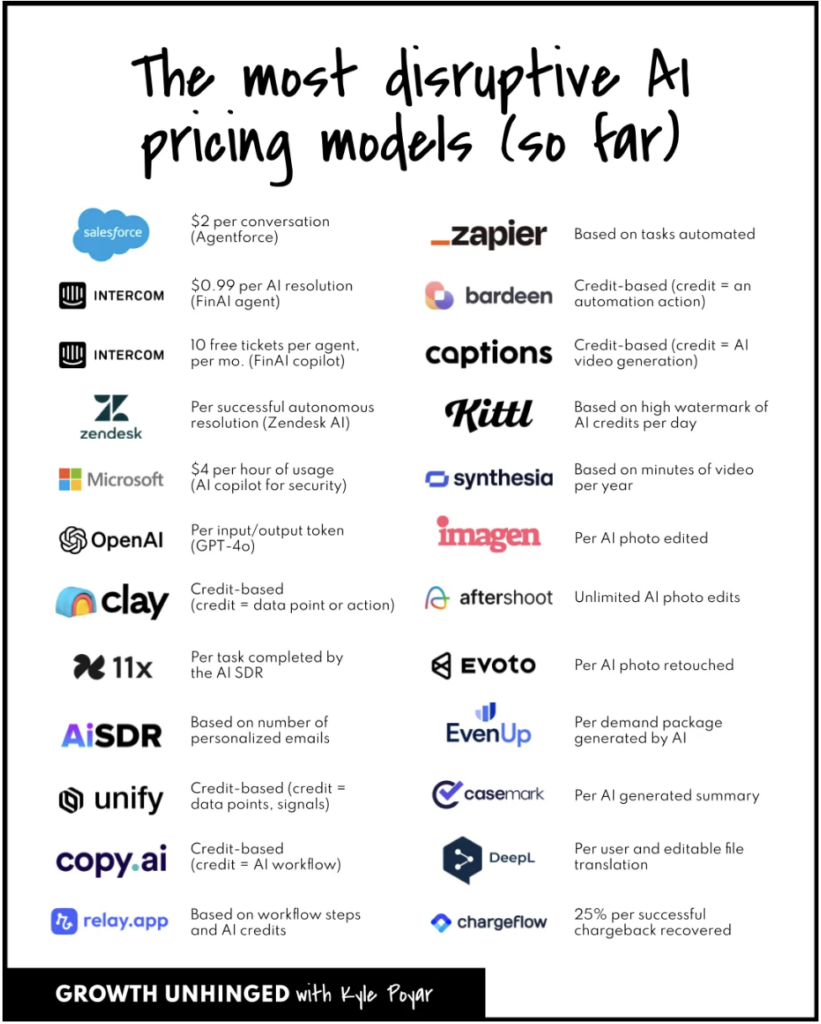
Implications for Investors:
Founders must be deliberate in designing business models that align with AI-driven cost structures and evolving customer expectations. Investors should look for early signals of pricing validation, such as evidence that customers are willing to adopt a usage- or value-based model at scale.
5. Does this company look like a VC or PE opportunity?
Because many vertical AI companies will look more like mini-agencies than traditional software companies (see point 1), we can expect some of them to behave more like PE—rather than VC—opportunities.
Given the level of consultative support required, and the limited differentiation across these AI solutions, many companies may struggle to scale beyond the 5-10m ARR mark. So while they may generate revenue sooner, they are unlikely to achieve the J-curve growth trajectory of traditional venture-backed software businesses. We may see many lookalike vertical AI applications spread across different geographies.
As a result, exits could happen sooner than in typical venture-backed scenarios, with PE-style roll-ups emerging to drive synergies across similar AI companies. This could resemble the consolidation of creative agencies under firms like WPP or Publicis Group.
However, some companies will break free from this low-differentiation agency model. The winners will be those that execute rapidly and expand their product vision with common horizontal use cases across multiple vertical applications.
A good example of this is Rippling, which identified that HR is built on top of many vertical applications, with duplication of functionality as each has their own security, permissions, integrations etc. Rippling unified these by using employee data as a central layer, and allowing vertical apps to plug into it. We expect similar winners to emerge in AI applications by “platformising” common operational use cases across the enterprise, unlocking network effects or proprietary data advantages. These businesses will look a lot more like venture outcomes.
Implications for Investors:
Early consolidation and in-organic growth levers are likely to have a bigger role to play for AI start-ups. Investors must have a high bar for product differentiation when backing category winners, or be comfortable with a potentially more capital intensive roll-up play for vertical specific AI applications.
While the early traction of AI companies is enticing, investors must be aware of the nuanced characteristics of these companies versus their SaaS equivalents. Understanding the technical depth of products as well as the appropriate business model, the role of services firms, and the appropriate growth strategies will be critical for AI investors.
At Frontline, we’re actively seeking visionary founders building enduring AI-first companies. If you’re tackling these challenges head-on, we’d love to hear from you. Reach out at [email protected].